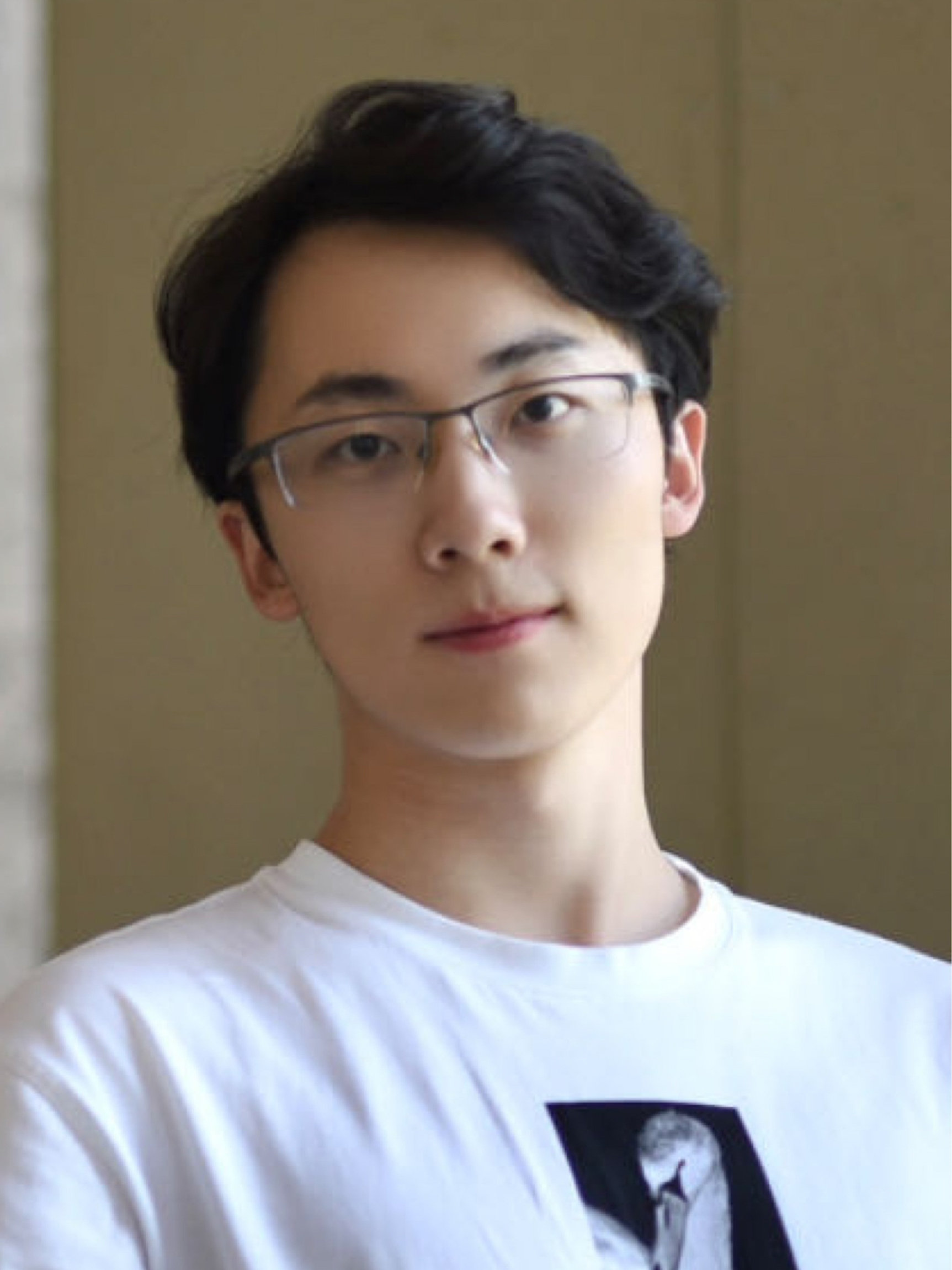
The first paper, “Graph Neural Networks Are More Than Filters: Revisiting and Benchmarking from A Spectral Perspective,” led by Dr. Yushun Dong, is accepted by International Conference on Learning Representations (ICLR 2025) and will be presented during the conference on April 24–28, 2025. This work challenges the conventional understanding of Graph Neural Networks (GNNs), revealing that the components beyond neighborhood aggregation—such as non-linear layers—significantly influence how GNNs process graph data across different frequency domains. This paper also proposes a novel benchmark to evaluate various GNN architectures from a spectral perspective, demonstrating the necessity of considering complete GNN structures in spectral analysis.
The second paper, “ST-FiT: Inductive Spatial-Temporal Forecasting with Limited Training Data,” co-led by Dr. Yushun Dong and Zhenyu Lei, is accepted by AAAI Conference on Artificial Intelligence (AAAI 2025) and has been presented during the conference on February 25– March 4, 2025. This paper proposes ST-FiT, a new framework designed to accurately forecast future dynamics on spatial-temporal graphs, even when training data is severely limited or unavailable for most nodes. ST-FiT introduces innovative temporal data augmentation and spatial topology learning modules, achieving superior predictive accuracy in real-world benchmarks compared to existing approaches.