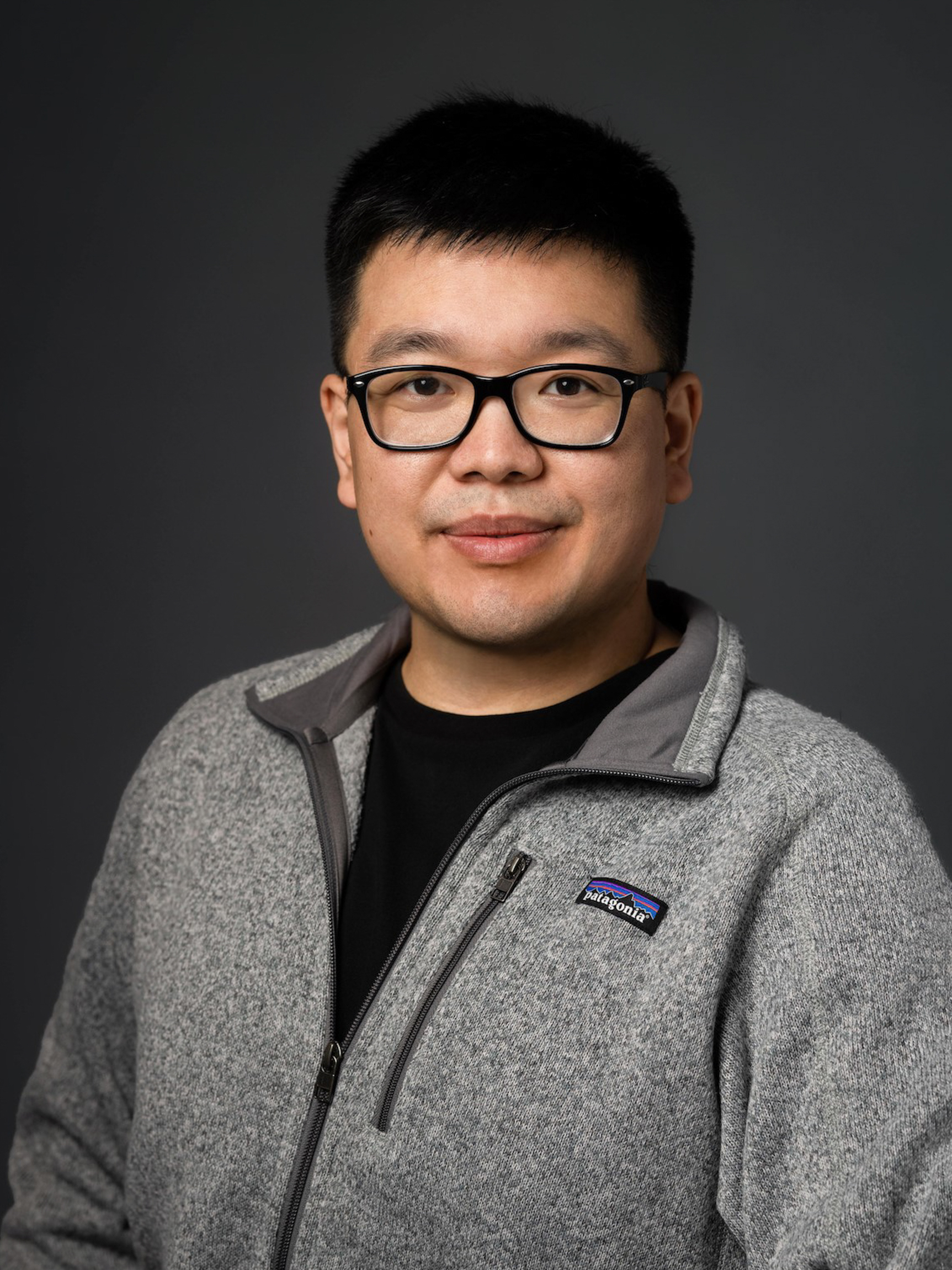
For the EMNLP 2024 paper:
Dr. Shangqian Gao of Florida State University has a paper accepted by the 2024 Conference on Empirical Methods in Natural Language Processing (EMNLP), the flagship venue for empirical NLP research. The paper is titled “Unlocking Memorization in Large Language Models with Dynamic Soft Prompting” and was coauthored by researchers from George Mason University, GE Healthcare, the University of Pittsburgh, and the College of William & Mary. Dr. Gao is one of the project leaders for this paper.
Large language models have transformed NLP by achieving breakthrough results in tasks like summarization, question answering, and translation. However, these models also pose significant security risks due to their tendency to memorize sensitive training data, which can lead to privacy breaches and copyright infringements. Theoretically, one would want to examine every possible combination of input prefixes and their corresponding memorized outputs—but the search space grows exponentially with the number of input tokens. Existing methods often rely on constant soft prompts that do not adjust to input variations, thereby missing opportunities to reveal hidden memorization.
To address this challenge, the authors introduced a dynamic soft prompting framework. Instead of relying on a fixed prompt, their method trains a transformer-based generator to produce adaptive, prefix-dependent soft prompts. This design allows the system to more effectively extract memorized data from the target language model. Compared to state-of-the-art approaches, their solution achieves up to a 135.3% relative improvement in discoverable memorization rate for text generation tasks and a 39.8% improvement for code generation tasks. The paper has been presented at EMNLP 2024 in November 2024.
For the NeurIPS 2024 paper:
Dr. Shangqian Gao of Florida State University has coauthored a paper accepted by the 38th Conference on Neural Information Processing Systems (NeurIPS 2024), the premier international conference for machine learning research. The paper is titled “DISP-LLM: Dimension-Independent Structural Pruning for Large Language Models” and was developed in collaboration with colleagues from Samsung Research America. In this work, the primary contribution comes from innovative techniques from Dr. Gao’s group.
Deploying large language models on resource-limited devices is challenging due to their enormous memory and computation requirements. Conventional structural pruning methods enforce a rigid layer-wise dependency—forcing all layers to prune the same feature dimensions—which limits flexibility and overall performance. Instead of following this one-size-fits-all approach, the authors propose DISP-LLM, a novel method that breaks these structural dependencies by applying index-based operations. This allows each layer to independently select its most informative subset of features along the embedding dimension without introducing any extra parameters. As a result, their technique not only preserves accuracy comparable to semi-structural pruning but also improves computational efficiency and adaptability. The paper demonstrates its effectiveness on models including OPT, LLaMA, and Phi. The paper has been presented at NeurIPS 2024 in December 2024.